NewYork-Presbyterian and Columbia cardiologists have developed the first artificial intelligence (AI) deep learning tool able to detect cardiac structural abnormalities associated with heart failure on chest X-rays. A new study led by Pierre Elias, MD, a cardiologist at NewYork-Presbyterian and Columbia and medical director for Artificial Intelligence at NewYork-Presbyterian, found that the AI tool is the first-of-its-kind to outperform radiologists attempting the same task. The technology could lead to the early diagnosis of heart failure.
“Most people with heart failure are diagnosed once they’re symptomatic, which we call Stage C,” says Dr. Elias. “But before that happens, there are structural changes to the heart, which we call Stage B. It thickens and dilates, undergoing what we call cardiac remodeling. So, we asked, ‘Could you use a chest X-ray to detect this?’ It turns out it is possible.”
A New Diagnostic Approach
To develop the deep learning model, Dr. Elias and his colleagues used chest X-rays and echocardiograms (ECHOs) from almost 25,000 patients to identify severe left ventricular hypertrophy (SLVH) and dilated left ventricle (DLV), structural changes associated with heart failure. The model extracted labels for SLVH, DLV, and a composite label indicating either abnormality from ECHOs performed within a year of the patients’ X-rays.
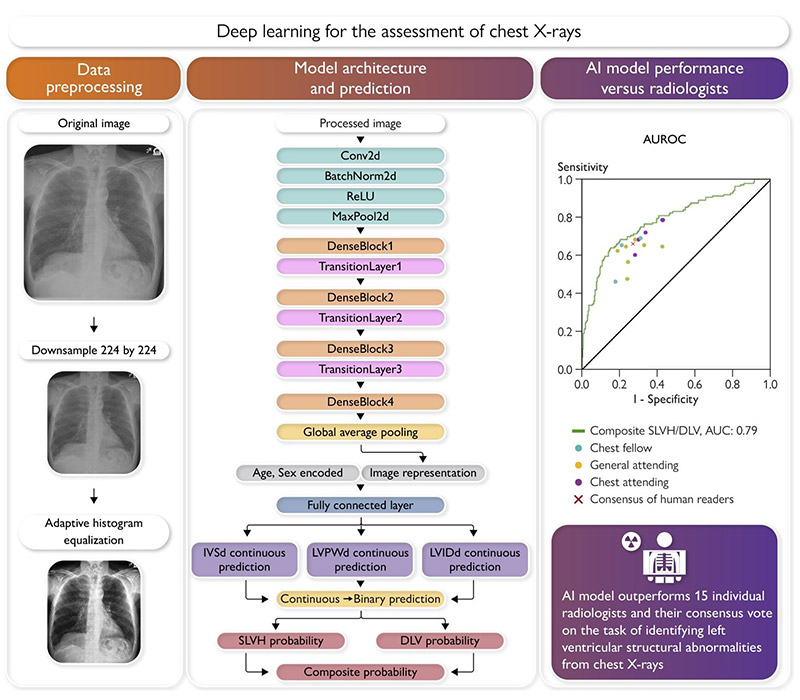
The deep learning model takes as input a pre-processed chest X-ray of dimension 224-by-224, age, and sex; the model outputs a probability for DLV, SLVH, and a composite label indicating the presence of either structural abnormality. (Graphical abstract from the European Heart Journal)
“We train our model to analyze the chest X-ray, while learning if a patient has SLVH or DLV using a more expensive but more accurate diagnostic test, specifically echocardiograms,” says Dr. Elias. “And then we see if we can exceed human expertise. The results support the idea that there is additional information on chest x-rays that doctors don't know how to interpret but that the model can interpret if you give it an accurate answer. That's what our lab focuses on and does.”
Most people with heart failure are diagnosed once they're symptomatic. But before that happens, there are structural changes to the heart. It thickens and dilates, undergoing what we call cardiac remodeling. So, we asked, ‘Could you use a chest X-ray to detect this?’ It turns out it is possible.
— Dr. Pierre Elias
The machine learning model accurately detected SLVH and DLV and was then validated externally using more than 8,000 chest X-rays from Stanford University. Lastly it was compared to readings by 15 board-certified radiologists.
Evaluated using area under the receiving operating characteristic curve (AUROC), the model yielded an AUROC of 0.79 for SLVH, 0.80 for DLV, and 0.80 for the composite label, with similar performance on the Stanford data set. The model outperformed all 15 radiologists for predicting the composite label and achieved a sensitivity of 71% vs. 66% against the consensus vote across all radiologists at a fixed specificity of 73%.
Dr. Elias says the model is roughly 7% more accurate than the radiologists. “To date, the best AI model was 90% as accurate as a human expert in the best case, and we have been concluding that AI models were inferior to the human expert,” he says. “Now we are saying, if you think about the paradigm in a different way, you can actually exceed human performance.”
Other AI Insights
AI is expected to change how clinicians obtain and review medical images in the next few years, and Dr. Elias’ lab is at the forefront of using AI to detect cardiac diseases earlier. His lab has also trained and tested deep learning models that can detect valvular heart disease from electrocardiograms (ECGs), potentially offering a simpler and less expensive option to echocardiograms. The resulting tool, the ValveNet ECG AI algorithm, accurately detected valvular heart disease in two independent clinical studies.
Similarly, an ECG-based deep learning model, EchoNext, detected structural heart disease in the largest validation of such a model to date. It could potentially be used to identify patients with undiagnosed disease in need of further assessment, such as echocardiography. The research is on-going.
The lab also trained a model using ECGs and echos to detect cardiac amyloidosis with similar accuracy in both Blacks and Whites, thus decreasing racial bias in diagnostic testing. Cardiac amyloidosis predominantly affects Blacks, who are diagnosed with more advanced phenotypes and experience worse outcomes than non-Black patients, yet are less likely to be referred for testing.
“It took years for me to start to believe that this can work,” says Dr. Elias. “There's still the question of how good it is, how good it’s going to be, and how much it might impact care. That's why we're doing these trials. But these models have now been run on hundreds of thousands of people, and there is growing evidence they work.”
Future Steps
Dr. Elias believes that the cutting-edge AI research is enabled by the people and infrastructure at NewYork-Presbyterian and Columbia. “You have to have access to data, and you also need to have people who support you and believe in this mission to go beyond traditional patient care. So that we can help provide not only the best of current care, but develop the future of care,” he says. “NewYork-Presbyterian evokes that in every possible way.”
To date, the best AI model was 90% as accurate as a human expert in the best case, and we have been concluding that AI models were inferior to the human expert. Now we are saying, if you think about the paradigm in a different way, you can exceed human performance.
— Dr. Pierre Elias
“We take care of an incredibly diverse patient population: Black, white, Hispanic, Asian, and every payer—Medicare, Medicaid, and private insurer,” he adds. “This allows us to build models that we think are fair, equitable, and work for everyone.”
The current study has Elias particularly intrigued by the potential that a simple chest X-ray holds for predicting disease. He considers the paper a call to action. “This is a ringing of the bell to say we need to do a few things. First, there's additional information on chest X-rays than we currently interpret, and we have to do a better job of interpreting them,” says Dr. Elias. “Second, we should be doing these investigations on the right populations to figure out if this an effective screening tool for detecting cardiac pathologies and early detections of heart failure.”
Many questions remain. “How do we educate radiologists to interpret more cardiac pathology on chest X-rays?” says Dr. Elias. “How do we find the right populations to assess this for screening tests, and how do we make this work in developing nations where there is potentially higher need and greater utility for using it?”
Fortunately, Dr. Elias says, his colleagues at NewYork-Presbyterian and Columbia are driven by the desire to further knowledge and forge new pathways toward better patient care. “We have a group of people who are at the top of their field, and are not afraid to say, ‘There are lots of limitations to the way we do things today. Let's do better,’” he says.
Dr. Elias and his colleagues are continuing to pursue research in this field, and recently published two additional papers on AI for cardiovascular care in the Journal of the American College of Cardiology – one reviewing the current state of AI applications in cardiology and the other looking at the promise and limitations of AI in cardiovascular care.